INTRODUCTION
Global public health concerns regarding the emergence of novel diseases have increased. The impacts of these novel diseases are often severe because the whole population is immunologically naïve to the emergent diseases, and medical treatments and vaccines are limited. In addition, the steeply increasing number of international travels between countries and continents, in the context of globalization, increases the pandemic risk by the emerging diseases. The coronavirus disease (COVID-19) pandemic is a prime example that shows how an emerging infectious disease (EID) can have a substantial impact on public health [1] and the world economy [2]. To make matters worse, the incidence of an EID is not a rare event. More than 300 EIDs have been reported from 1940 to 2004 [3] and the emergence of infectious diseases such as Hendra, Nipah, Severe Acute Respiratory Syndrome (SARS), Middle East respiratory syndrome (MERS), etc., is still an ongoing challenge that needs to be tackled [4, 5].
Early response is one of the key strategies to minimize the impact of EIDs at the local and global level. Relevant evidence of the benefits of early response have been reported for Ebola [6] and Influenza [7], and it has also been accumulating for COVID-19 in various countries [8]. According to a recent study [9], a three-week delayed response could have caused 18-fold more cases, and a three-week earlier response would have reduced 95% of the cases during the initial phase of COVID-19 in Wuhan, China. In enhancing the capability of early response against EIDs, typical approaches have been the identification of the potential zoonotic pathogen in wildlife [10] or identification of high-risk areas for spill-over events (i.e., the transmission of pathogens from animals to human population) [4, 11]. Considering that about 60% of EIDs are transmitted from animals [3], these efforts could motivate local health authorities to increase preparedness for EIDs or to facilitate the processes of vaccine development before the pathogen encounters the human population.
Strengthening the surveillance capacity against novel infectious diseases or EIDs could be another approach to enhance the capability of early response. Surveillance system with high sensitivity (i.e., detecting disease events based on small number of cases reported), and with high timeliness (i.e., shortening the time gap between occurrence of the events and detection), could initiate interventions in the early phase of the events [12]. In this regard, identifying regions with poor surveillance capacity is important to prioritize areas for the improvement of surveillance system.
In this study, we aimed to provide a prediction map to suggest regions with low surveillance capacity for novel infectious diseases. Specifically, we focused on capacity of internet-based surveillance systems. Although there are several limitations (e.g., heterogeneity of internet access between regions or countries [13]) in using internet-based disease surveillance systems, such systems are gaining popularity as a tool for early detection of epidemics before an outbreak is officially recognized, which initiates epidemiological investigations [14, 15]. Indeed, the SARS outbreak in 2002 demonstrated the potentials of the early detection capacity of internet-based surveillance systems [16]. In the present study, we specifically targeted surveillance capacity for unexplained death events as a proxy for the surveillance capacity on novel infectious diseases. The unexplained death events were defined as a human death case from a suspicious infectious disease without a confirmed diagnosis in the first report. Therefore, only one death could be included. Using unexplained mortality cases rather than morbidity cases, we can minimize potential bias from characteristics of diseases (e.g., severity) when measuring regional surveillance capacity.
MATERIALS AND METHODS
A global-level study was conducted in three steps. First, reports of unexplained death events from 2015 to 2019 (5 years) were collected from the most commonly used web-based surveillance systems, and relevant information, such as geographical locations (i.e., countries, states or districts) and indicators of surveillance capacity (i.e., sensitivity and timeliness), were extracted from the reports. The two indicators for surveillance capacity were the main outcome variables. Second, potential predictor variables for the surveillance capacities for unexplained diseases were collected for all global regions, except for Antarctica. The predictor variables included demographic, socioeconomic, public health, and geographical factors. The study unit was a one by one-degree latitude-longitude grid covering the world (N = 17,666). Third, machine learning algorithm-based prediction models were developed using the extracted surveillance capacity indicators and predictor variables in each grid where the unexplained death events were reported. Subsequently, predicted risk values for lower surveillance capacity were produced for every study unit using the extracted predictor variables in the grids and the developed prediction models.
The reports for unexplained death events were collected from the two internet-based surveillance systems, ProMED-Mail [17] and the Global Public Health Intelligence Network (GPHIN) [18]. ProMED-Mail uses information from media reports, official reports and local observers. The collected reports are then reviewed by analysts or experts before being disseminated to subscribers or published on a website. GPHIN incorporates natural language processing methods to systematically collect information from news articles, media releases and incidence reports and to categorize the information based on pathogen type or hosts etc.
In order to collect reports of unknown diseases from ProMed-Mail, we designed a search string including the terms “undiagnosed OR mysterious OR mystery OR novel OR unknown.” For the purpose of gathering reports from GPHIN, we developed a search protocol with the following inclusion criteria: 1) reports published in English only, 2) reports containing at least one type of infectious disease, and 3) titles containing “unknown” or “undiagnosed” or “mysterious.” The exclusion criteria for both data sources were as follows: 1) non-first reports, 2) novel subtypes of diseases (e.g., novel strain of norovirus, novel influenza), 3) prion diseases (e.g., posts labeled “novel prion update”), 4) endemic diseases, 5) unknown sources (e.g., unknown source of food-borne infection), and 6) components (e.g., unknown component of drugs).
Two indicators reflecting the surveillance capacity, sensitivity and timeliness, were defined as follows: 1) sensitivity—the number of mortality cases at the first report, and 2) timeliness—the time gap (in terms of days) between incidence of mortality cases and reports (Supplementary Fig. S1). As the target outcome of this study was unknown diseases, various types of diseases could be included with different levels of symptom severity. Considering that the varying levels of symptom severity may affect the evaluation indicators independent of surveillance capacity, only reports with mortality cases were included in this study.
Considering that quantitative investigations of associated factors of surveillance capacity are still lacking, we assumed that a comprehensive range of potential factors, including regional, demographic, socioeconomic, public health, and geographical factors, could affect the surveillance capacity for unknown diseases. The data for the variables were acquired from various sources (Table 1).
Category | Variable | Data source |
---|---|---|
Demographic | Population | NASA SEDAC [19] |
Socioeconomic | Night time light level | NASA [19] |
GDP | Kummu et al. [22] | |
Human development index | Kummu et al. [22] | |
Income based country classification | World Bank [2] | |
Public health | Health expenditure | World Bank [2] |
Geographic | IHR score | World Health Organization [1] |
Urban land use | Tuanmu et al. [26] |
Demographic variables were obtained from the fourth version of the Gridded Population of the World (GPW v4) [19]. GPWv4 is a raster format global-scale data with a resolution of about 1 km. As the data included estimated population sizes in years 2000, 2005, 2010, 2015, and 2020, average values for the two recent years, 2015 and 2020, were used for the analysis. Night-time light levels, regional gross domestic product (GDP), and human development index (HDI) were used to represent the regional socioeconomic status. Night-time light level data was satellite-based remote sensing data acquired from the National Aeronautics and Space Administration’s Black Marble night-time light product [20] which was a raster-type data with a 500 m resolution. Higher night-time light levels were assumed to be associated with higher levels of regional economic activities [21]. GDP and HDI were acquired from a published report by Kummu et al. [22] in which the annual raster-type data for GDP and HDI were provided with a resolution of approximately 10 km for the period 1990–2015. Considering the period of this study (2015–2019), only data of 2015 was used in the prediction models. In addition, income-based country classification by the World Bank (i.e., high-income, upper-middle-income, lower-middle-income, and low-income) [23] was included as a categorical variable. National-level variables representing the level of public health system performance or surveillance capacity were also used, such as health expenditure [24] and the average of 13 International Health Regulations (IHR) core capacity scores reported by the World Health Organization [25]. Urban land use was used as a geographical factor. The land use data were acquired from Tuanmu et al. [26], which provided a consensus dataset of land use converging four global land cover products: DISCover, GLC2000, MODIS2005, and GlobCover. Among 12 land use types including forest, water and agricultural land, we considered only urban land use, which is known to be associated with accessibility to medical facilities.
The acquired predictor variables with spatial features were preprocessed to calculate values for each study unit grid. Crop and mask functions were used for clipping the raster data to fit into each grid and the getValue function was used to extract values where raster cells intersected with each study unit grid. The functions for the preprocessing were obtained from the raster package [27] in R v.4.0.2 [28]. The geographical distribution of the acquired variables was shown in Supplementary Fig. S2 and S3.
Boosted regression tree (BRT) [29] was applied to predict the risk of low surveillance capacity for unexplained deaths on a global scale. As a tree-based machine learning method, BRTs incorporate non-linear associations into high-order interactions between variables and usually produce better predictability than traditional generalized linear models. Previous studies have conducted global-level prediction using the BRT method, but the predictions were for other types of outcome variables (i.e., incidence of emerging zoonotic diseases or antimicrobial resistance) [4, 30]. Although BRT is limited in that it is considered a type of black-box technique and the effect of each predictor on the outcome variables cannot be quantified, relative influence of each variable can be determined. Considering that we used two indicators for measuring surveillance capacity (the number of mortality cases at the first report and the time gap between incidence of mortality cases and reports), two prediction models were fitted, one for each indicator (Model 1 for sensitivity and Model 2 for timeliness). Specifically, two binary outcome variables were used: 1) whether the number of mortality cases in the first reports were equal to ten or higher (Model 1); and 2) whether the time gap between the occurrence of mortality cases and reporting were equal to one week or longer (Model 2). Leave-one-out cross validation was used with area under the curve of the receiver-operator characteristic (AUC of the ROC) to validate predictability of the models. Because the previous global level prediction studies using BRTs produced an AUC of 0.67 [30], we did not determine the predicted risk for low surveillance capacity if our prediction model showed an AUC of less than 0.67.
RESULTS
An initial search retrieved 2,276 and 658 reports of unknown diseases from the two internet-based surveillance systems, ProMed-Mail and GPHIN, respectively. After examining relevance by screening the title and contents of each report and removing duplicates from the data sources, a total of 327 reports remained. Out of the 327 reports, 198 (60.5%) reports included human diseases with and without mortality cases. The remaining 129 reports showed mortality cases only and thus were used for analysis. Out of the 129 reports, a majority (104 reports, 80.6%) were from low-income or lower middle-income countries. Among the 129 reports, 14 did not provide detailed location information other than the country name, and 28 reports did not contain information on the time gap between the occurrence of death and reporting (Fig. 1). The geographical distribution of reported unexplained death events with an assessment of the surveillance capacity by the two indicators are shown in Supplementary Fig. S4.
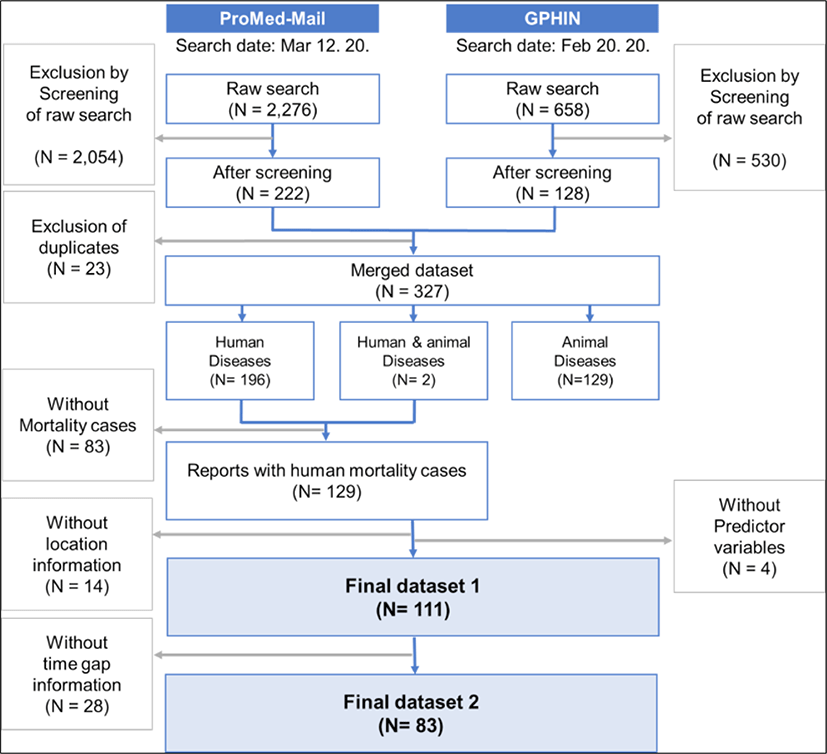
Results of univariable logistic regression analysis, shown in Tables 2 and 3, provide an overview of the associations between the predictors and the two indicators of the surveillance capacity. In terms of the number of mortality cases shown in the first report, IHR score and all socioeconomic variables, including natural log of GDP per capita, national income-based country classification, natural log of HDI, and night-time light level, showed significant negative associations (Odds Ratios [95% confidence intervals] were 0.758 [0.604–0.918], 0.076 [0.004–0.384], 0.038 [0.005–0.236], 0.973 [0.945–0.995], and 0.970 [0.951–0.989] for natural log of GDP, income-based country classification, natural log of HDI, night-time light level and IHR, respectively). However, none of the associations were significant for the time gap between the occurrence of mortality cases and reporting.
Low sensitivity was defined as the number of unexplained death cases in the first reports were equal to ten or higher.
Income-based country classification by World Bank (4 categories; high income, upper middle income, lower income, low income). The odds ratio was for high and upper middle-income levels compared to low and lower middle-income levels as a reference.
Poor timeliness was defined as the time gap between the occurrence of mortality cases and reporting were equal to one week or longer.
Income-based country classification by World Bank (4 categories; high income, upper middle income, lower income, low income). The odds ratio was for high and upper middle-income levels compared to low and lower middle-income levels as a reference.
The results of the two prediction models using BRT are as follows: The LOOCV AUC was 0.70 (indicating a moderate validity) in Model 1, but 0.58 (indicating a rather low validity) in Model 2. Socioeconomic or public health-related predictors (night-time light level, health expenditure, HDI, IHR score, and GDP per capita) showed a higher relative influence than the others (Supplementary Fig. S5). As low AUC values were obtained in Model 2, we predicted risk of low surveillance capacity by using Model 1 only (Fig. 2). The averages of predicted risk of low surveillance capacity were 45.2%, 37.4%, 12.5%, and 3.0% in low-income, lower middle-income, upper middle-income, and high-income countries, respectively. In terms of geographical classification, the sub-Saharan African countries showed the highest average predicted risk (43.0%) and North America showed the lowest average predicted risk (2.7%).
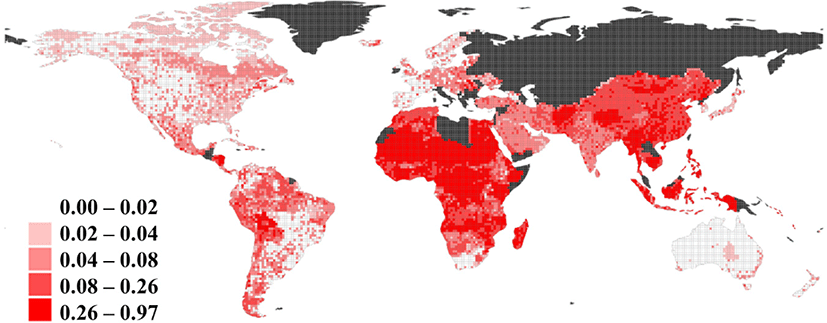
DISCUSSION
The purpose of this study was to develop a prediction map representing the risk of low surveillance capacity for unexplained deaths in a global scale, in order to prioritize regions for strengthening surveillance capacity. To this end, we acquired reports of unexplained death events from internet-based surveillance systems and various predictor variables including demographic, socioeconomic, public health, and geographic factors. Surveillance capacity was measured by two indicators; the number of mortality cases during the first report (sensitivity) and the time gap between occurrence of mortality cases and reporting (timeliness). Two prediction models were fitted, one for each indicator, but only the model for predicting sensitivity showed reasonable validity, revealing a high risk of low sensitivity in low income countries and the sub-Saharan region.
The findings of this study, based on the outcomes from the logistic regression models and BRT results using sensitivity measurements, suggest that socioeconomic and public health-related factors can explain the risk of lower sensitivity for unexplained death events detection. The clear differences between regional socioeconomic statuses could be attributed to the availability of different levels of human and financial resources for surveillance by the regions. High disease burden with higher and more frequent mortality events in resource-poor regions could also explain the results. Considering that the risk of novel disease emergence is also high in the low-income and lower-middle-income countries [4], the results imply the urgent demands for improving early detection and response capability in the risk area.
On the other hand, the lack of predictability of Model 2, which was fitted to timeliness measurements, indicates that the current predictor factors are insufficient to explain the timeliness capacity of the surveillance. Latent predictors, which are associated with timeliness but were not used in this study, or a lower sample size than that used for Model 1 could contribute to the lack of predictability. However, the results could also indicate that internet-based syndromic surveillance systems function appropriately in low socio-economic areas, compared to the others, in terms of timeliness. A previous study that evaluated timeliness capacity for reporting of EIDs showed that the time gap between disease onset and report tend to be high in African countries [14]. However, the findings of our study suggest that contrasting results could be derived, presumably, due to the following differences between the studies. First, the previous study used symptom onset to measure timeliness, not incidences of death as used in our study. Second, the time period of the previous study was between 1996 and 2009, but we used data between 2015 and 2019. Third, the target outcome was a WHO-verified outbreak in the previous study, but we employed unexplained death as the target outcome.
In addition, we found that only 18 out of 129 unexplained death events were reported to both ProMed-Mail and GPHIN, suggesting low agreement between the two data sources. The low level of agreement may imply that multiple data sources should be incorporated for practical implementation of internet-based surveillance systems for unknown diseases.
This study has several limitations. First, our study only evaluated the sensitivity and timeliness of internet-based syndromic surveillance systems. We recommend follow-up studies to assess other attributes such as data quality, cost-effectiveness, predictive value positive, etc. [31] and to evaluate other types of syndromic surveillance systems, which use hospital-based clinical data usually obtained from emergency departments [32]. Second, our analysis could not incorporate any temporal variations; previous studies have suggested that surveillance capacity could be enhanced over time [14]. Third, we included only two internet-based surveillance system in spite of the existence of many other data sources such as Healthmap, Medisys, etc. Incorporation of more data sources of internet-based systems will improve the data quality for this research.
Despite the limitations, to the best of the authors’ knowledge, this study is the first to conduct a global-level prediction for low surveillance capacity specifically targeting unexplained death. Our results suggest that enhancing surveillance capacity is particularly important and needed in sub-Saharan Africa and in low-income countries. Recently, the World Bank initiated the West Africa Regional Disease Surveillance Systems Enhancement Project, which aims to strengthen capacity of infectious disease surveillance in West Africa [33]. However, the early detection capacity still needs improvement [34], especially for surveillance sensitivity, as revealed in this study.